This Privacy Policy describes how we collect, use, protect, and disclose your personal information.
Collection of Personal Information
We may collect the following types of personal information:
a) Identity Information: Name
b) Contact Information: Email address, phone number
c) Demographic Information: Occupation
d) Preference and Feedback Information: Product or service interests, opinions, and suggestions about our products or services
e) Activity Participation Information: Records and interaction data from your participation in our events
We may collect your personal information through the following means:
a) Event registration forms
b) Event feedback forms
c) Customer satisfaction surveys
d) Online and offline interactions
e) Social media interactions
f) Phone or email communications
Use of Personal Information
We may use your personal information for the following purposes:
a) Conduct market research and analysis
b) Provide or improve our products and services
c) Contact you to provide relevant information, offers, and promotional activities
d) Respond to your inquiries and provide customer support
e) Comply with applicable laws and legal requirements
Protection of Personal Information
We implement appropriate technical and organizational measures to protect your personal information from unauthorized access, use, or disclosure.
Disclosure of Personal Information
We may share your personal information in the following circumstances:
a) Affiliated Companies: With our subsidiaries or affiliated companies for the purposes described in this policy
b) Service Providers: With third-party service providers that assist us in our business operations
c) Business Transfers: In the event of a merger, acquisition, or sale of assets
d) Legal Requirements: Disclosing data as required by law
Retention of Personal Information
We will retain your personal information for the time necessary to fulfill the purposes for which it was collected unless a longer retention period is required or permitted by law. The retention period will consider:
a) The purpose of data collection
b) Legal, accounting, or reporting requirements
c) The need to resolve disputes or enforce agreements
Your Rights
You have the right to:
a) Understand how we process your personal information
b) Obtain a copy of your personal information that we hold
c) Request correction of inaccurate or incomplete personal information
d) Object to the processing of your personal information
e) Obtain a copy of your personal information in a readable format
f) Request the deletion of your personal information or withdraw your consent at any time
Policy Updates
We may update this Privacy Policy from time to time. Any changes will be posted on our website, and the effective date will be updated accordingly.
Contact Us
If you have any questions, concerns, or complaints about how we handle your personal information, or if you wish to exercise your rights, please contact us at info@netron.net.
認識AWS Kendra
Kendra是什麼?
Amazon Kendra 簡單來講是 Amazon Web Services (AWS) 提供的一項企業搜索服務,它是使用機器學習來提高搜索結果的準確性和相關性。
Amazon Kendra 可以在各種內容存儲庫中找到答案,包括文檔、網頁、電子郵件和社交媒體。
大型語言模型(LLM)的幻覺
但造成這種情況的原因是宇宙神秘力量嗎!?並不是!其實是因為有些AI的訓練數據比較老舊,因而有機率無法進行有效的規劃跟整理,所以就沒有辦法回答使用者,但是AI還是想要吐給使用者一個答案,進而造成這種我問它A,結果它回答我B,這種牛頭不對馬嘴的情況發生。
檢索增強生成RAG
為了要避免上述的這種情況發生,我們就需要用檢索增強生成,也就是俗稱的RAG,所謂的RAG(Retrieval Augmented Generation)就是透過提示工程推理,再加上LLM,雙管齊下,丟給我們的AI整理好的資訊,讓它能好好的針對問題回答。
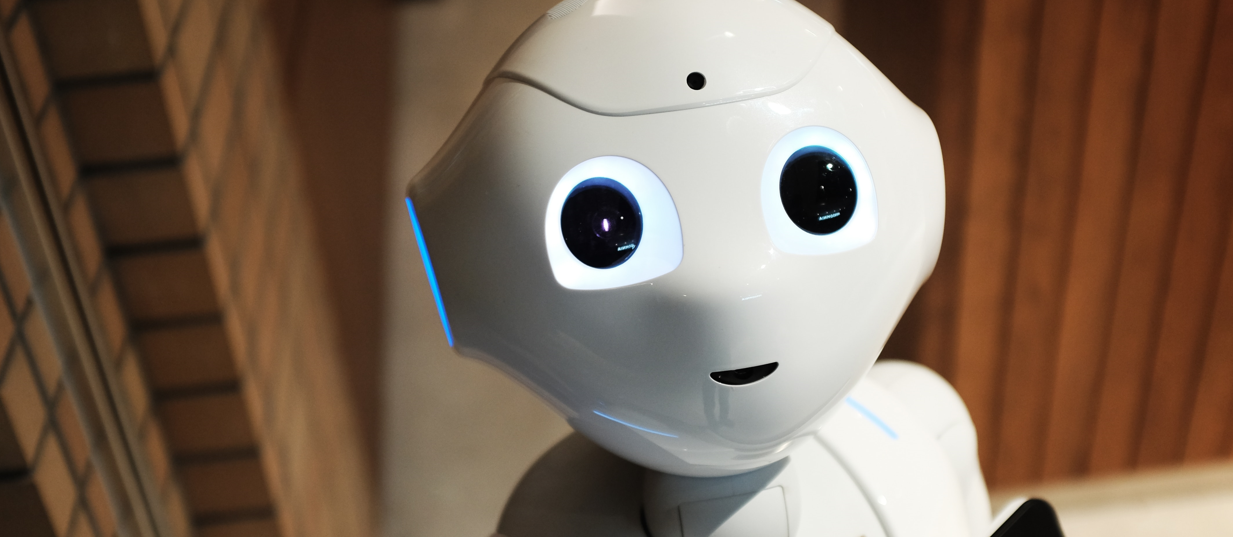
目的就是讓AI根據提供的資訊直接的回答問題,不要創造對話中未包含的內容,確保LLM精準的回答問題本身,不要再讓AI當編劇啦!
搜尋引擎 Kendra
所以我們知道,要讓LLM不要亂回答問題,重點是要餵給它正確的內容,來人!餵公子吃餅!(威~~~ )既然要餵正確的資料,所以搜尋引擎就變成了很重要的角色,因為它需要理解使用者的問題,找到相對應的答案之後再丟給LLM去找答案
這邊就要介紹我們今天的主角「AWS Kendra」。
Kendra它具有以下幾個特性:
1. 支援自然語言以及關鍵字查詢
蝦米!?自然語言?是有關自然的語言嗎?當然不是,自然語言指的口語化的內容,就像是人類彼此正常交談一樣,讓你跟Kendra溝通時,可以像是跟人類說話一樣,用口語化文字讓Kendra去查詢相對應的內容;關鍵字查詢就很好理解了,大家常常在使用網路找尋資料,我們想找資料時所輸入了隻字片語就是所謂的關鍵字,舉個例子,我想要知道今天台北的天氣如何,用自然語言查找我就可以輸入:「今天台北的天氣如何?」,用關鍵字查找就可以輸入:「台北 天氣」。透過這種口語化的發問方式就能取得理想的結果。
2. 基於NLU和ML搜索
因為Kendra擁有NLU(Natural Language Understanding)這樣的特性,在搭配上ML(Machine Learning),Kendra會透過
顧名思義Kendra閱讀資料後理解文件內容。
針對使用者常常對Kendra下的問句或是關鍵字查找的內容。
最相關的內容排名順序。
Kendra會根據以上的這幾點去進行結果的分析,將搜尋引擎找到的內容做一個收斂,並且拋出最適宜的內容給使用者或是給LLM。
3. 支援多種數據來源
Kendra作為一個搜尋引擎,最重要的是可以多方攝取資料,而Kendra當然也可以做到這點,它就像隔壁棚的名偵探X南一樣,在於查找方面鐵定是不放過各種蛛絲馬跡,因為Kendra支援超過50種的流行資料來源。
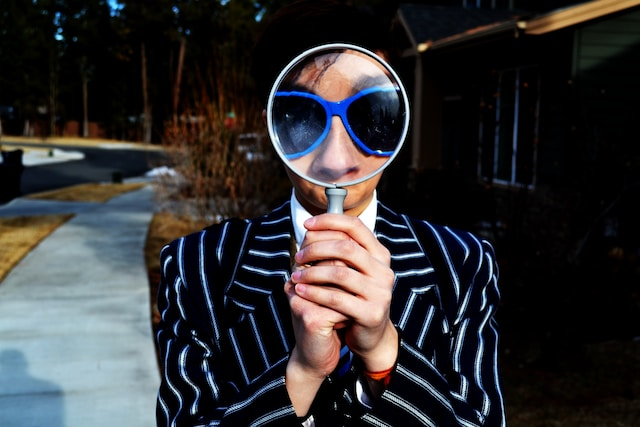
4. 針對領域進行最佳化檢索
我們常常聽到一句話叫做術業有專攻,Kendra針對14個主要領域進行了優化,讓我們在使用Kendra這個搜尋引擎的時候,只要涉及到這些範疇內的內容可以更好的找到答案。
這14個主要領域分別是:IT、金融服務、保險、製藥、工業、能源、法律、媒體和娛樂、旅遊和飯店、健康、人力資源、新聞、電信和汽車。
5. Kendra新特性
Kendra除了上述4點之外,還有一個酷酷的特性,就是它可以針對「自訂義文件豐富化」。Kendra這個新特性可以允許使用者在數據攝取期間,透過自訂義的攝取管道,在文件被Kendra查找到之前,先進行所謂的「預處理」。
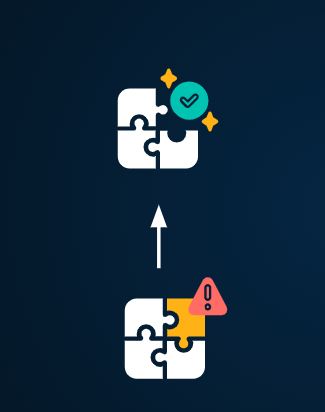
什麼是預處理?其實也很好理解,就是預先處理的意思,舉例來說,如果我們提供Kendra查找的內容,有包含了一些資料敏感的個人資訊,我們就可以透過自定義文件將這些資料先一步進行清除,等到Kendra找到這些資料的時候,已經是不含這些資料敏感內容的版本了,這就很好的幫助我們將資料進行第一步處理;除此之外這部分也可以調用Lambda函數,或是對於圖像進行光學符號的識別,或對文本進行翻譯等等…。
下一代搜索的標準架構
現在我們已經比較清楚Kendra是個什麼樣的搜尋引擎了,由此延伸出來,為了達成RAG的模式,所以下一代的搜索架構就會是由搜尋引擎加上大型語言模型。
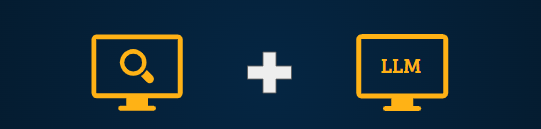
由搜尋引擎藉由使用者所下的指令,負責找到最精確的內容,以及過濾掉更多的有害物質。再由大型語言模型以更加人性化且自然的方式,進行資訊的閱讀,最後再將答案展現到我們的面前。
套用到各行各業
這時候我們其實就會有一個疑問產生,雖然好像被筆者吹成這樣,但是你我真的用的到AI服務嗎?這個答案我想是肯定的,我們都知道下個世代絕對是AI的時代,很多東西都可以透過AI來協助我們完成,而且各個領域也有全然不同的且大量的Domain,以傳統的方式來說,我們將以人工的方式找到相對應的資料庫,搜尋期間就會耗費不少的時間,以不見得每次都可以找到相對應的資訊跟答案。找到資訊之後,我們仍然要花費大量的時間去閱讀並且理解內容,況且也不見得能夠找到理想中的答案。但是這個過程又是不可避免的。
時間拉回現在,我們可以藉由這種好用的工具來協助我們完成,並省去大量的時間,對於我們產出會有十足的幫助。
從架構來了解
簡易的架構該如何組成呢?大致上分為幾個步驟:
1. 使用者從前端的Web介面下達指令後(通常是輸入想要獲取的資料關鍵字或自然語言),
2. 通過AWS的API Gateway去向AWS Lambda發出請求,
3. 會調動AWS的Kendra這個搜尋引擎去找出最符合使用者想要得到的資料內容,
4. 再來把這些資料再餵給LLM,讓LLM根據這些內容去找出或是彙整出答案,並回答使用者,
5. 最後把這些提問的紀錄存放在AWS DynamoDB,作為歷史紀錄。
而使用者也可以透過資料放置到S3 Bucket,成為可以被Kendra搜尋到的Datasource。

結語
希望透過今天簡單的介紹,可以讓大家對於AWS Kendra有更進一步的了解,也可以知道我們該如何確保LLM能不要亂回答啦!如果大家也想要利用Kendra還有LLM幫助我們提升工作效率,或是想要知道AI會如何簡短我們工時,也歡迎各位聯繫我們網創資訊啦!今天的介紹就到這邊結束囉~~~ 大家掰掰!